Top-5 Cost Reduction Engineer Challenges of Today
Today’s cost reduction engineer must take the lead in integrating a design-to-cost (DTC) approach into product development cycles. The DTC approach helps enterprises cut design costs early on to avert late-stage redesigns that may delay product launch dates. Simply put, this cost-focused methodology enables organizations to capture savings while maintaining the functionality and quality of their product designs.
However, there are five major pitfalls that would prevent cost engineers from implementing a DTC strategy across a product team. These five challenges also jeopardize the collaboration, coordination, and productivity among colleagues in a costing department.
In this article, we discuss the role of a cost-reduction engineer in implementing a cost-focused approach within an organization. We also examine the five major challenges that cost engineers are currently facing, as well as how to overcome them.
What is a Cost Reduction Engineer?
The cost reduction engineer is a costing specialist that assesses and determines the cost of many new product designs. They leverage a target cost approach to ensure that products are optimized for cost and manufacturability right from the start. The target cost approach requires cross-functional collaboration among departments within a product team to successfully meet cost targets.
Using digital twins, a cost reduction engineer can accelerate product costing and make more informed costing decisions. The digital twin replicates tangible products through virtual 3D CAD models stored in product life cycle management (PLM) systems. Digital twins can be integrated with an automated manufacturing insights platform, such as aP Pro, to modernize product cost analysis.
According to McKinsey & Company, cost teams that use digital twins can influence 50 percent faster time to market (TTM). The cost reduction engineer can also work with designers to improve the quality of new products by 25 percent. These figures reaffirm the importance of equipping cost engineers with advanced software to streamline their cost analysis process.
Five Major Challenges to a Cost-Reduction Strategy
There are five major obstacles that are currently impacting the cost-reduction strategy of modern cost engineering teams. In addition to impacting their performance, these manufacturing challenges prevent cost engineers from fostering a cost-conscious environment inside the product team. The five major challenges include:
1. Slow Product Cost Reduction Methodologies
Some costing departments fail to recognize that identifying potential savings opportunities is only one aspect of their job. They must also share cost insights with each area of the product team to capitalize on these cost-saving opportunities.
To ensure that savings are captured quickly, the cost reduction engineer cannot waste time performing manual cost estimates. Traditional cost analysis methods, such as spreadsheet software, are prone to error and difficult to manage.
Cost engineers need a manufacturing insights platform to improve the accuracy of their cost estimates and to achieve the following:
- Automate and accelerate the cost analysis process using real-world manufacturing conditions.
- Assist the sourcing team in locating reasonably priced components or materials from global suppliers in today’s inflationary market. Materials can include more sustainable alternatives that designers can use to design products.
- Align senior leadership’s cost and manufacturability targets with internal colleagues across all functional areas.
2. Inadequate Inter-Team Collaboration
Poor cross-functional collaboration prevents internal functions within a product team from obtaining the cost insights they need. It is a challenge for functional areas to collaborate with each other when they work in silos or use disparate technologies. When cost insights are siloed and not shared with the entire product team, the following outcomes may occur:
- Sourcing managers are unable to get a fair price for components without should-cost estimates. The sourcing department needs should-cost projections to initiate fact-based negotiations with suppliers and achieve savings on components.
- Designers jeopardize cost-effective product development when they consider using parts that are not locally available and have hidden costs. It is crucial to identify the macro-level factors that may drive up the cost of reasonably priced components. For instance, Bloomberg reports that the current inflationary episode has increased logistics costs by 47 percent beginning in January 2021.
Costing expertise must be shared by the cost reduction engineer across all product team functions, including design and sourcing. Cost teams require a manufacturing insights platform to assess how current price increases may affect the cost structure of key components. They can also use the platform to find discrepancies between the current cost of components and generated accurate should-cost estimates.
3. Failing to Consider the Total Cost of Ownership
The total cost of ownership (TCO) of a product includes the purchase price as well as operating expenses. Some cost teams prioritize only direct material expenses and disregard all other costs associated with a product’s life cycle.
By neglecting TCO, cost engineers overlook the critical and ever-changing costs of key areas like logistics, labor rates, and tooling. The cost reduction engineer needs a manufacturing insights platform to track all costs associated with the entire product development process. The simulation-driven platform leverages the following to generate accurate, end-to-end product cost estimates:
- Real-world regional data libraries. Cost engineers can produce estimates based on actual labor rates across the globe. Regional data also includes indirect overhead rates and inputs, machine overhead rates and inputs, tool data, SG&A, and more.
- Real-world manufacturing cost models. Teams can use mechanistic cost models to simulate manufacturing processes and calculate associated costs. Some simulation outputs include process cycle time calculations, tooling costs, material utilization, and manufacturability analysis and guidance.
4. Uncertainty About How Frequently Product Cost Analysis Should Be Performed
Some manufacturing firms may regard product cost analysis as an occasional task for cost engineering teams. However, because the real-world manufacturing environment is always changing, the cost team must frequently assess the cost of various products.
Cost engineers need the right skills and manufacturing software to stay on top of disruptions that could affect product costs. Senior leadership must provide cost teams with resources, such as digital technology, that foster competitive advantage and transform cost-reduction strategies. At its core, senior executives must ensure that cost engineers have the tools they need to do their jobs efficiently.
5. Resistance to Cultural Change
When cultural change is introduced into a company, it is common for some personnel to be resistant to those changes. The same is true for the cost reduction engineer attempting to instill a cost-focused culture across an entire manufacturing organization.
For instance, the fourth industrial revolution (Industry 4.0) has streamlined manufacturing processes by introducing automated digital technologies to enterprises. This includes a manufacturing insights platform, which automates cost analysis and gives stakeholders access to cost data in one place. Although Industry 4.0 modernizes manufacturing operations, McKinsey reports that only 48 percent of manufacturers feel prepared to adopt advanced technology.
Cost departments require the influence and support of senior leadership to bring internal colleagues on board with a cost-focused culture. Leaders must convey the value of digital technology in breaking organizational silos and aligning DTC goals across the product team. A manufacturing insights platform, for example, can increase cross-functional productivity by 26 percent through collaborative data sharing.
Conclusion
Cost engineers encounter ever-changing obstacles that affect their performance and ability to influence colleagues to incorporate cost into their functions. To successfully introduce a more productive, cost-focused culture to colleagues, cost teams require digital manufacturing insights and top management backing.
How to Build a Modern Costing Department
See how to organize and develop a costing team to get products to market faster and with greater profits.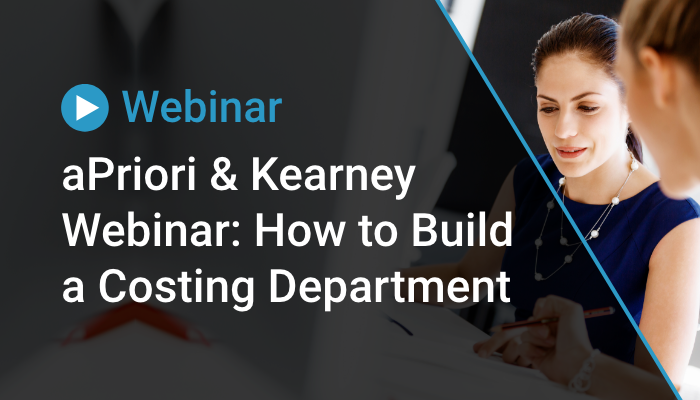