November 3, 2022
How Will AI and Machine Learning Impact Manufacturing?
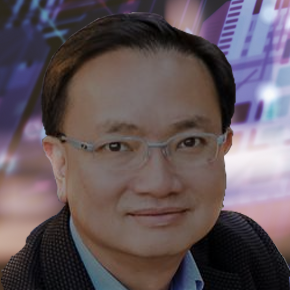
Transcript
You may not know it yet, but manufacturing is transforming due to the impact of artificial intelligence and machine learning. My guest today, Christopher Nguyen, is the CEO and co-founder of Aitomatic Incorporated. He is one of the world’s leading advocates for AI engineering. For the last four years, Christopher has spearheaded the global AI effort at Panasonic, working to implement machine learning in manufacturing, avionics, energy, and automotive. Today, we are here to talk about the future of AI and machine learning in manufacturing. Christopher Nguyen, welcome to the podcast.
Christopher Nguyen: Thank you, Leah. Thanks for having me.
How Is Artificial Intelligence Used in Manufacturing Today?
Leah Archibald: Can you tell us how AI is being used in manufacturing today?
Christopher Nguyen: Well, the somewhat flippant answer is not enough. [chuckle] There’s so much opportunity.
Leah Archibald: That’s a good answer. How is Artificial Intelligence being used today? And how should it be used in manufacturing?
Christopher Nguyen: Or how it could be used? I think in terms of potential, you can think of it as multiple layers. You can think of the bottom layer as the actual equipment. And then the process management layer, and even higher at the product management cycle – how people are making decisions using data and AI.
Leah Archibald: So maybe you need to explain to me a little bit more how the AI works. Because when I picture AI, I kind of think of a black box that’s doing things. I don’t know what it’s doing, but it’s hopefully coming up with the right answer.
Christopher Nguyen: The analogy I like to use – and every analogy has some imperfection – is human intelligence doing pattern matching. If you think about that analogy, you understand perhaps 90% of what’s needed to capture the essence of what AI and machine learning technology can do for us. We are constantly doing pattern matching. We would not exist as humans if we could not do pattern matching. We learn from patterns, and then based on those patterns, we see new things and we slip them into pattern. Sometimes quite incorrectly, which leads to discriminations and stereotypes and so on, but for the most part, they work reasonably well. So AI has all of those properties and limitations as well. This pattern matching is being used in cybersecurity for manufacturing. Today plants are pretty much a connected system, so there’s bits and bytes flowing around. Equipment’s talking to each other, they’re talking to control systems and so on. So over the course of a week, if you were to watch the network traffic, you will see that it has certain ebbs and flows and patterns. And then one day if there’s a cyber attack, somebody coming in with a strange packet of information, you would recognize that. You would say, “Hey, this is strange.” Kind of like a strange person coming to a party. You don’t have the guest list, but this person clearly doesn’t look like they belong. You say, “Well, this is an anomaly, something is wrong.” So that’s an example of how best to understand at a very high, but a very effective level, what AI is and what it can do for us.
Leah Archibald: In terms of cybersecurity, the AI is watching for a pattern that maybe doesn’t fit and that’s how it can recognize a security threat. What about in the manufacturing technology itself? Is there a place for artificial intelligence there?
Christopher Nguyen: Let’s take the same concept of detecting patterns. One major application that’s being rolled out is defect detection. You’ve got thousands of parts coming through, they all look exactly the same, and then there’s one that if there’s an IC chip, one leg is standing up. A human will recognize that, but not at that speed or not at that level of reliability. Maybe you can put an AI algorithm in there to look at that and say, “Hey, something is different about this.” And maybe if it’s smarter, it can say, “This is what’s wrong.”
Only 10% of Manufacturers Take Advantage of Artificial Intelligence
Leah Archibald: And what percentage of manufacturers would you say are adopting AI technology? Before you said not enough, but could you take a stab in the dark to give us a sense of how much of the industry is affected already?
Christopher Nguyen: I would estimate no more than 10% globally.
Leah Archibald: Is it because these systems are very expensive to implement or overly complicated?
Christopher Nguyen: That’s actually a much deeper question. Let me share with you an explanation. It sounds somewhat roundabout, but I’m a Silicon Valley guy. When my last company was acquired by Panasonic, I took on this responsibility to implement AI across the global footprint of Panasonic. It was 250,000 people. It took us months to figure out that manufacturing moves at the speed of atoms, whereas Silicon Valley moves at the speed of bits. It’s much easier to move information than it is to move things. All this is to say that there is a reason why adoption of AI is low in manufacturing. It’s not because there aren’t a lot of very smart people involved. It’s just that manufacturing has to move at a certain pace. And you’re right, it does cost more. In the oil and gas industry, one piece of equipment that is down for one hour costs $100,000 an hour. It’s not the piece of equipment itself, it’s the economic impact of the downtime.
Leah Archibald: So there’s probably a lot of fear around adopting AI systems, even if they are going to save you a lot of time and money in the future in terms of how fast they could speed up your assembly line. It sounds like getting over the hump of doing the adoption for manufacturing is a big hurdle. So how do you propose that manufacturers do get over that initial hump of adoption?
Christopher Nguyen: First of all, now that I’m a little bit smarter than I was four years ago, I wouldn’t use the word fear. I think it’s a very reasonable concern for the most part. When you put a bunch of very smart people in the room, given this problem, they would still move at that speed because of the constraints of the problem.
Leah Archibald: I want to hear about one of these demonstration projects that you did at Panasonic.
Christopher Nguyen: The first one that we did took us the better part of a year to figure out how to do it in the physical world. Panasonic is very involved in what’s called a cold chain: how a fish gets from the ocean fresh to your dining table. Clearly, there’s refrigeration everywhere in the transport in between, and Panasonic makes a lot of that equipment.
Leah Archibald: Because if at any point the fish gets too hot, then it’s wasted, right?
Christopher Nguyen: Right. And if people get poisoned or something, then there’s a lawsuit. So increasingly the validated supply chain becomes more important. People are actually willing to pay more if they can see the providence of the produce that they’re buying. So one piece of equipment that is always present in these refrigeration systems are the compressors and the filters. And these things, when they fail, can be easily replaced, but as you imply, it’s the knock-on effect that really matters. So the state of the art is preventive maintenance, right?
Leah Archibald: Being able to predict which compressors are gonna fail.
Christopher Nguyen: Well, yes. That’s the holy grail: predictive.
Leah Archibald: It was so easy for me to say, why can’t your team do it?
Christopher Nguyen: [laughter] It turns out, digital machine learning, as it is today, cannot solve that problem. And the reason for that is very simple. Machine learning, as I said earlier, is pattern matching. In order to predict these failures, therefore, you must have a pattern of failures. Meaning, you must have a lot of examples of these things.
Leah Archibald: Which you don’t want to have.
Christopher Nguyen: Exactly. [laughter] So catch-22. With machine learning, what we can do is what’s called “anomaly detection.” That’s the party example that I gave earlier. You invite a bunch of people, 100 guests coming to your house party, somebody tried to crash, and they’re dressed differently –
Leah Archibald: You say, “There’s something about that guy. It’s just not right. I can’t put my finger on what, but it’s just not right.”
Christopher Nguyen: Right. So now you can identify the anomaly, but you cannot identify the fault. Those are two different things. So we basically solve half the problem. We can alert. We can say, “Hey, something looks different about this compressor, or these compressors today.” Now it’s up to the human operator to figure out what to do with that information. So that turns out to be not a complete solution. Because actually it can be quite costly. The compressor may behave differently simply because the workload is different. And so now if that was false alarm somebody has to create a new workflow for people to check it. So it became very necessary for us to solve the second half. And that’s something that we cannot yet do with database to machine learning, because you and I just talked about the fact that there is no data for that purpose. So insight the light bulb went up when we said, “What if we just incorporate the knowledge of the operators?” Because they can easily look at a compressor and say, “This is fine.” Or they can say, “Oh yeah, if I look at the pressure and the temperature at that point, the compressor looks like it might fail.” So if we can capture that domain expertise, that human knowledge, and then put it into this AI system, now we have a real predictive system.
Can Artificial Intelligence Solve the Labor Crisis in Manufacturing?
Leah Archibald: A lot of executives I’ve talked to on the podcast are not specifically interested in AI, but they are interested in capturing human knowledge in their models. So a lot of manufacturing companies are investing in having a digital thread, where they have a model of their particular component, and it goes through a digital factory so they can predict which machines are going to be more cost-effective.
Christopher Nguyen: That’s right. Conceptually, these things are very comprehensible, but it’s the tooling that is getting more and more advanced in realizing what you and I are talking about here.
Leah Archibald: So you’re trying to incorporate the siloed knowledge of the human beings and build them into the model, because that’s really the rate determining step.
Christopher Nguyen: Yes, that’s exactly it. And in fact, our tagline is: “Our software translates human expertise into machine learning models for industrial companies.”
Leah Archibald: This is what so many execs have been telling me: that the biggest challenge other than supply chain issues is labor – finding skilled labor.
Christopher Nguyen: That’s right.
Leah Archibald: It’s training skilled labor, upskilling people who maybe don’t have those skills. So this is a different way of thinking about the solution. As opposed to, “How do I get more people?” You’re saying, “How can I maximize the labor pool I already have by bringing in artificial intelligence to complement them with what they’re doing?”
Christopher Nguyen: Right. And that’s a perfect way to describe the long-term picture as well. I like to push a term called “IA”, Intelligent Augmentation, as opposed to AI.
Leah Archibald: IA, Intelligent Augmentation. I like that. So let’s go back to the manufacturing executive who perhaps is hearing this for the first time, and wondering how they could onboard AI into their company. How could they be intelligently augmented in their role?
Christopher Nguyen: What we did at Panasonic can be instructive as one template, which is to think of it as a two-prong effort. There’s a six-month effort and there’s a six-year effort. The six-month effort is to identify low-hanging fruits that you can use as demonstration project. Because companies are social organizations. You want to demonstrate success, and then people will follow that success. So do not go with the biggest most challenging project first. Do something that will affect maybe a group of 10 or 20 people. They will become the evangelists, and make the awareness factor. But to do that as an organization, the commitment has to be from the top. I ran Gmail at Google where the budgets were very important. With those initial budgets, you have to assume that small AI projects are investment projects. You cannot use a normal P&L to measure those things. You’ve got to look at the effective long-term P&L.
Leah Archibald: The executive has to be really forward-thinking. Not just, “How is this going to solve a problem for me now?” but “How is this going to solve the problems of labor and of supply chain instability and the problems that I don’t know about yet? How is it going to solve those problems six months, six years, or 60 years into the future?”
Christopher Nguyen: That’s right.
Leah Archibald: Christopher Nguyen, thank you so much for joining me on the podcast today.
Christopher Nguyen: Thank you, Leah. It’s been enjoyable.
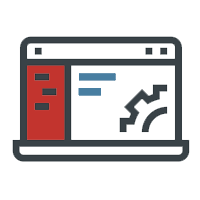