Accelerate Your Product Cost Analysis With Bulk Costing
Utilization of traditional product cost analysis methods has been replaced with automated, smarter ways to estimate product costs. Today’s cost engineers can assess the cost of multiple parts at once using manufacturing simulation software with bulk costing capabilities. Bulk costing produces fast, detailed business insights that assist cost teams in identifying cost-cutting opportunities across all product designs.
In this post, we will discuss how cost teams can streamline the product cost analysis process with bulk costing. It also details how automated costing allows teams to produce more accurate estimates based on different scenarios and real-world factors.
What is Product Cost Analysis?
Product cost analysis defines the processes and tools used by cost engineers to assess the cost of new product designs. The cost analysis process considers all the costs associated with manufacturing a product from infancy to launch.
Cost analysis was once entirely manual, but with the rise of automated and simulation-driven software, it has been streamlined. Cost engineers can now estimate product costs automatically and spot cost reduction opportunities in the early stages of product development. These insights can be used by the entire product team to optimize their products or parts for cost and manufacturability.
What is the Importance of Bulk Costing in the Product Cost Analysis Process?
Today’s cost engineering teams simply do not have the time to assess the cost of thousands of product parts. The number of new product designs they receive from a large design team limits their ability to provide timely estimates. This challenge is exacerbated when cost teams use manual, time-consuming spreadsheets to produce estimates that are prone to human error.
Cost teams may use a bulk costing tool to produce quick and accurate cost estimates to keep pace with new designs. Bulk costing is an automated module in manufacturing simulation software such as the aP Pro platform. It enables cost engineers to improve the efficiency of their product cost analysis by:
- Generating fast and detailed cost estimates for multiple parts at the same time.
- Identifying expensive outliers for cost reduction opportunities.
- Providing insight into whether parts will be easy or difficult to manufacture.
How Does the Bulk Costing Module Work and Locate Areas for Cost Reduction?
Cost engineers can start employing a bulk costing tool by simply using the digital twin with 3D CAD design files. They can then access a digital twin file directory for product lines, commodity groups, and new product development (NPD) projects. It allows users to quickly select and cost all the existing designs stored within a digital twin file at once.
Cost teams gain access to a top-level view of a specific project once the tool has generated its cost estimates. Teams can use data visualization to discover outliers in parts where cost reduction is possible. They can even see the entire cost breakdown of a component by clicking on its outlier data. Some of the insights provided by this view include:
- Labor costs
- Material costs
- Direct overhead costs
- Indirect costs
The wealth of information provided by bulk costing allows the cost team to extend its influence across departments such as:
- Sourcing. Cost specialists can identify gaps between the current price of components compared to a should-cost projection generated by the module. The sourcing team can leverage these should-costs to begin fact-based discussions with suppliers and achieve savings on key components. This is especially important when negotiating savings in the current inflationary environment, as rising material costs drive up supplier prices.
- Design. Engineers gain insight into design outliers for parts that are most challenging to manufacture. The module delivers these insights from both a design to cost (DTC) and a design for manufacturability (DFM) perspective. Design teams can collaborate with cost engineers to quickly act on the manufacturability and cost issues of a new design.
What is Matrix Costing and How Does It Simulate Different Manufacturing Scenarios?
The matrix costing capability is another key component of the manufacturing bulk costing module. Matrix costing workflows can be launched to estimate the cost of one component or product using multiple production inputs.
At its core, matrix costing allows cost teams to easily and quickly simulate different manufacturing scenarios in a single workflow. Below are three production inputs that the cost engineer must set to run a simulation with matrix costing:
- Locations. Users can simulate the production of their parts in different areas of the world. Matrix costing considers all the changes to regional economic variables such as labor rates and other real-world factors.
- Annual volumes. Users can specify the minimum number of products they would like to produce each year.
- Batch sizes. Users have the option of splitting product batches in two ways: annually and quarterly.
The cost team’s different production combinations are all automatically evaluated by matrix costing to produce detailed cost reports. Cost analysts can use these reports to compare each simulation and determine which scenario has the lowest fully burdened cost.
These insights can be leveraged by the sourcing team to determine the most cost-effective regions for sourcing a component. They can also discover which global regions, based on supplier capacity and speed, are optimal for expanding their supply base.
Conclusion
Bulk costing has transformed product cost analysis with an automated and simulated way to assess the cost of new designs. Cost engineers can use bulk costing to quickly uncover cost-cutting opportunities and influence cost-first design and sourcing decisions. The ability to automate product cost analysis drives the potential of the entire product team to dramatically improve their results.
Get Closer To Your Actual Product Costs
Our Cost Estimation Guide Shows You How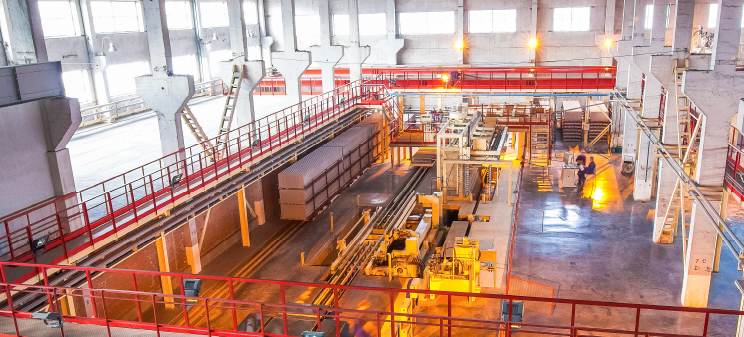