Video
Eaton: Digital Engineering Tools & The Future of Product Development
Embark on Eaton’s transformative journey in the realm of digital engineering, as revealed in this insightful video presentation. Witness the demystification of AI’s role, the integration of cutting-edge digital engineering tools like aPriori, and the visionary roadmap for the future.
Explore how Eaton navigates the challenges through model-based systems engineering and leverages artificial intelligence for intelligent automated design. Delve into the convergence of authoritative data sources, connected infrastructures, and scalable computing to revolutionize digital engineering at Eaton.
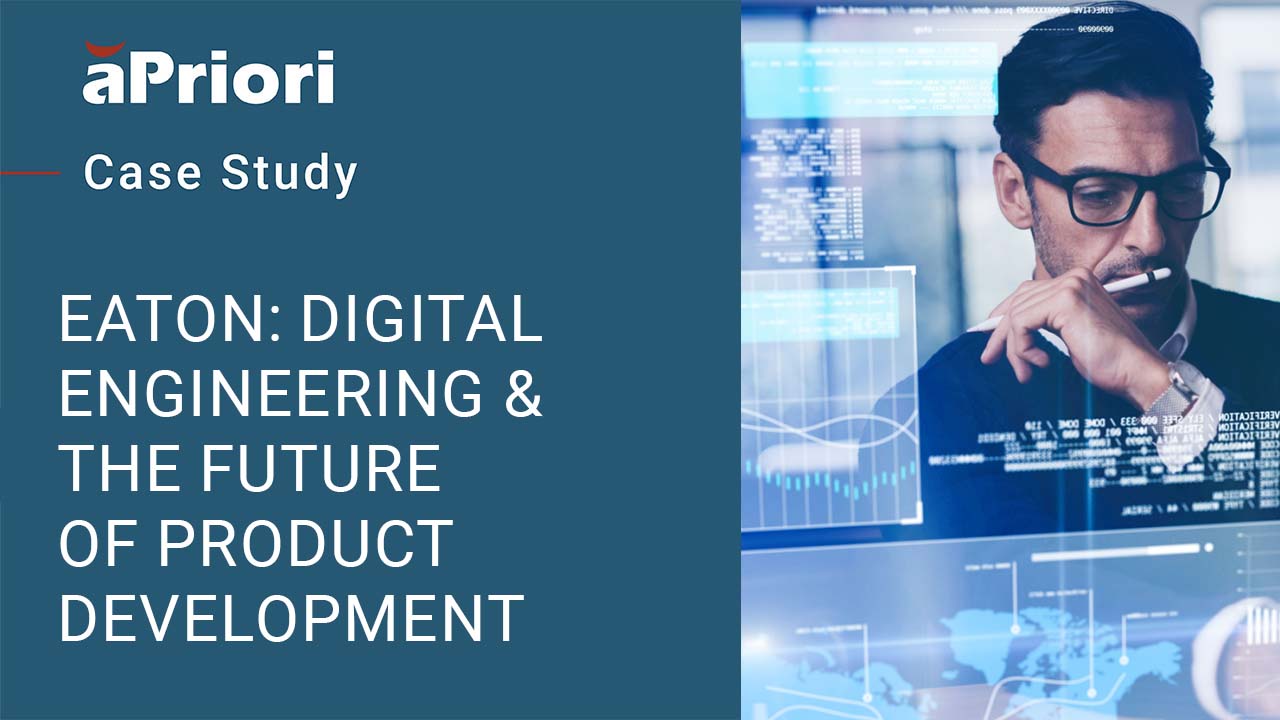
Transcript
Thank you for having me here. It’s a great honor to be here and standing in front of you. And we just had two great presentations, right? And Stephanie talking about why we need to change. We had Peter Zeihan giving a very broad overview of why change is coming. And today I’m going to level set you on what’s happening at Eaton as well, in terms of this change in digital transformation.
So who are we? Today people need a couple of things to do what they do, they need transportation, they need technology, most of you have your phones on you. You had to get a means to get here. We need energy, right? Look around us. And all these technologies are what we do at Eaton. Eaton is a power management and technology organization. And we have over 92,000 employees across 175 countries, $20 billion organization. And if you think about what we do, we make what matters work. And that’s what I just shared with you. Right? Look around you, everything that matters to you, we’re into it.
Eaton’s Digital Engineering Strategy
So from managing energy to your components, to your systems, to your products in your manufacturing plants, managing the power consumption as well of those solutions, that’s what we do across electrical and industrial sectors. In our electrical sectors, we’re looking at power distributions, we’re looking at contract automation. And for our industrial sectors, we’re looking at aerospace components, we’re looking at e-mobility and vehicle components as well. So our digital engineering strategy really comes from the top down.
A quote from our CEO Craig Arnold, his statement in one of his newsletter this year, he says, “To capitalize on the opportunity ahead of us, we must execute flawlessly.” And our CTO, Raja Macha doubles down on this and says, “We need to double the outputs of Eaton’s new product innovation investment towards zero lead time.”
I teach product management in Industry 4.0 at Rice University, and I teach the need to specify metrics and visions, and when you provide the metric “zero lead time”, what does that mean to you as a metric? Can we really measure zero? And that’s what I’m going to try to convey to you today.
The Future of Digital Engineering
So as a product digitalization or digital transformation manager, my role is to take that vision and methodology and apply it to how we create products. And the vision from our VP of Engineering, it says, “Hey, Eaton design me an X.” Today you can say, “Hey Siri set an alarm for 5:00 AM.” You can say, “Hey Siri, close the garage door.” Right? So the future we’re looking at towards is one where ur customers can say, “Hey, Eaton design me an X.” And I’m gonna walk you through an example here.
So meet Bob, that guy. Bob works as a design engineer at ATC Models. And today, Bob, it takes five months for Bob, from when Bob reaches out to us to ask for a design of a standard valve stem, to when he gets a drawing, in terms of the cost, what it’s gonna take to actually create it. So that’s five months of lead time. And what I’m gonna show to you is the future we envision at Eaton where Bob is gonna connect with our digital engineering and digital design infrastructure either through a phone call or going on our app to say, “Hey, can Eaton design Bob a standard valve stem?”
And it’s gonna go like this. So Bob is gonna make a call and say, “Hey Eaton, can you design a standard valve stem for passenger car application?” And the response from our system is going to say, “Yeah, sure, we can do that. Is this for intake or exhaust system?” And Bob goes ahead and says, “Yes, this is for exhaust. I would like to prioritize the cost, I would like to prioritize the weight, I’d like to prioritize the lead time.” The system then takes this information and says, “Well, if you just told me to design a standard valve stem, what other information do I need to design a standard valve stem?” And the system is intelligent enough to ask and say, “Yes, give me some parameters here. Specifically, I need the information about the head diameter, I need information about the stem diameter, I need the seal diameter, I need the overall length.” And these are inputs that are coming from our customers today when designing a standard valve stem.
Digital Engineering, Automation, and Artificial Intelligence
Bob responds with the information for those parameters and the system says, “Sure.” We’re at the aPriori conference and it’s very important today, more than ever, to take the cost criteria into consideration, to take the lead time, to take the geographic location where this part is going into consideration when designing the part.” So the system is going to prompt Bob and say, “Hey, what’s the quantity that you have in mind and what’s your target price, if you have any? What’s the range of price? What’s the location where this is going?” Bob provides this and says, “Well, I need 6000 pieces, I need it at about 20 bucks each, it’s going to a customer in Austin, Texas.” And the system responds and says, “I have a couple of design options for you, I’ll send you the top five.” This is a vision that we have at Eaton in terms of digital engineering, and that’s going to take our traditional design processes from months to really minutes and even fraction of minutes.
So the output of that conversation that I showed you where we are today is our ability to take a query like that and automate design and cut down up to 95% in the turn around time and provide the user’s information including the materials, the weight, the cost, and how long it would take to deliver this product, really minutes as opposed to months. So the example I just gave you, what’s under the hood? And that’s what I’ll spend some more time to discuss with you this morning.
First I’d like to demystify AI. AI is going through the hype cycle, it’s not just a buzz word anymore, it’s not just the hype that every CEO and CTO says and uses in their discussion, but we are actually seeing it in use today. However, within the engineering and manufacturing community, what’s the limitation of AI? So I felt the need this morning to walk you through the scenario I just presented and walk you through how ChatGPT, specifically ChatGPT 3.5, which is the version that’s online and free today and everyone can use, and how it responds to a query like this when it comes to engineering design and manufacturing.
So I went online and I said, “Okay, ChatGPT,” I logged in and I said, “Well, design a standard valve stem for a passenger car application.” And through its nature, it responded and said, “Absolutely.” Right, and went on to highlight 10 key elements that I need to design a standard valve, specifically the materials and the length and all the things. But what it did not do was give me a drawing or a product. And to its credit, it actually did a good job identifying those 10 and they made complete technical sense. The next step I prompted further, ChatGPT is a very good AI solution, you can have a conversation with it, and I said, “Well, can you give me a drawing, a model, an image of what this looks like?”
Can AI Generate Engineering Drawings?
And it says, “Well, I can’t really provide you one, but think about a shiny product.” And went on and provided all that. And the question is still, can AI today create engineering drawings? So I took a step back and said, “Well, ChatGPT,” I said, “Well, is there any AI solution out there today that can create me an engineering drawing based on prompts? So effectively can AI generate engineering drawings and high fidelity engineering products?” And then the response of ChatGPT, it says in our reading here, it says, “The level of detail… ” First it says there’s advancement in AI, specifically generative adversarial networks. That’s really leading AI capabilities. It says, there are advancements there in its ability to generate very good images, however, when it comes to engineering design, and I’m gonna read it here, it says, “The level of detail required for precise engineering specification may not be fully achieved today using AI.
It says, “For engineering design… ” This is the last paragraph there on the screen. It says, “For engineering design, it is advisable to rely on computer aided design, CAD software and the expertise of human designers and engineers.” So effectively, the oracle has spoken, it says, “Hey, go rely on CAD designers and rely on the CAD software to create high fidelity engineering design.” So if you ask me today, and if I go back to the example I gave you and we say, “Well I lead a digital engineering group and we’re doing digital engineering and we’re able to show the example I provided at the beginning, what’s truly under the hood? What are the key digital engineering principles Eaton uses? There are five pillars that I wanna present to you today.
Authoritative Source of Truth
The first is establish an authoritative source of truth. Yes, we’re building AI capabilities to automate. And you already know why based on Stephanie and Peter’s presentation this morning. Right? But these algorithms need a place to go get engineering data. ChatGPT for example had Wikipedia and other sources of data where it could generate its intelligence. However for engineering, for manufacturing, where does AI get its data? So for an organization like Eaton we have to establish an authoritative source of truth where algorithms and workflow and automations and individuals as well can get the data they need to design products more efficiently.
Digital Engineering via Connected Infrastructures
The second is creating infrastructures that are connected. Can I connect my simulation software to my CAD software, to my DFM software like aPriori to our PLM solution, where we store and manage engineering… and drawings, to SAP, which is our ERP system. Can we have a connected infrastructure so that we can build automation across this infrastructure? And that’s the second.
Model-Based Engineering
And the third one here is model-based engineering. Based on the response from ChatGPT that I showed you, it says it cannot create high fidelity drawings and models. It’s not intelligent enough to run simulations today. It doesn’t have the code, it doesn’t have the knowledge.
Using Digital Engineering Tools to Model Manufacturability, Cost, and Sustainability
So what we’re doing is we still rely on those high fidelity simulations, we still rely on CAD models and drawings and tools like that that help us create engineering drawings. So what we’re doing instead of traditional design processes that does not look into extensive modeling and simulation, for us to truly do digital engineering, we need to rely on modeling. And that’s where tools like aPriori comes in, where we can model manufacturability, we can model cost, we have tools that we can use to model structure, we can model CFD and fluid transfer and thermal loads and so on.
Artificial Intelligence Needs to be Data-Driven
The fourth is what we call intelligence automated design. This is really where AI comes in. AI can come in and sit on top of a lot of simulation of data and starts to have inference on what parameters have the most impact on the output of interest. AI can also sit on top of a process where we’ve defined the best way to design a product, and we can logically define that process programmatically and have an algorithm run through it. Right? So that’s where intelligent automated design comes in.
And the last here is our computing infrastructure. We need computing infrastructure that’s scalable, that can grow with our needs and our customer’s needs. So here we need Cloud computing, we also need computing on the edge, we also need servers within our network that will handle computational need, based on our customers and data security policies. So effectively, a hybrid computing infrastructure is the last pillar here that’s helping us drive digital engineering at Eaton.
So if I give you an example of just three of this pillars, so I combine here the infrastructure, connected infrastructure, an authoritative source of truth, and here you’re seeing Ansys, Enovia, Volta, storing our simulation models, modeFRONTIER helping us with optimization. We have Abaqus, we have Matlab, and so on. Right? Mathcad. And we have Python as well today as an engineering tool. Our ability to streamline and connect to all of this. And for example I’m showing aPriori and what aPriori does for us as one of the tools that we’re using and how it connects with all the tools that we’re using.
How Eaton Uses aPriori
So we’re using aPriori for design to cost. Being able to identify and eliminate cost drivers early on in our design processes. We’re using aPriori for should-cost negotiation, our supply chain is doing that extensively. We can use aPriori to automate internal quoting when we get requests from our customers and we need to quote based on our digital factories that we’ve set up, we use aPriori for design for manufacturability, to look at manufacturability issues in our drawing, early on in the design phase.
And the last one which I really like is our ability to cut across traditional manufacturing processes as well as new manufacturing processes like additive manufacturing and the variations. There are so many ways or methods for additive manufacturing today, and how can we look across all of that and pick the one that is most suitable for the product and also meets our customer’s needs.
Model-Based Systems Engineering
So the next example I’ll give you here is model-based systems engineering. So for the parts, for standard valve stem, and this is an actual example of what it takes to design an end-to-end standard valve stem. We need to look at the deformation of the parts, we need to look at stresses in critical locations. And these are engineering models that we build. We need to look at the fatigue of the valve, we need to look at thermal response of that product. We have engineering calculations and rules that we have to look into and fully digitize. And again, you have aPriori there where we’re using aP Pro and also using the AP on the cloud as well to look at the cost, the manufacturability. And currently we’re integrating this to look into sustainability as well. So for model-based engineering, what we’re doing is representing the critical to quality elements of our design and making sure that we can represent them using models.
Digital Engineering Tools and Artificial Intelligence
So after that, we can pull all of this together, we can pull the infrastructures and we can pull the models and then create a process, how do we create this part today manually and code that into a workflow? In our workflow, I’m giving you an example here, we’re able to look at material selection and then code that into a workflow structure, design calculations. We’re looking at analysis of the fatigue behavior of our products, we’re looking at cost and lead time using aPriori. And once we build this workflow, we can run it multiple times, putting 100 design data into it and get outputs. And then start doing optimization of that using tools. And where AI comes in here is once we have 50 data points, 100 data points, that are based out of our stimulation in this workflow, we can put AI on top of this and create reduced-order models. Instead of this workflow running in 12 hours, in 13 hours, our reduced-order models literally run in seconds, and in some cases, some fraction of seconds. With a good enough fidelity of its prediction.
And once we have these results, the next thing is that we can take this AI algorithm that it gives us, deploy it on our cloud infrastructure, and the example I gave you becomes realistic, right, where you put in your inputs, it runs very quickly, iteratively running through thousands of design iterations and coming up with a five-top design. Those five top-designs are then fed through a high fidelity simulation to make sure that what the AI is giving us and the design we end up with is with good fidelity.
Generative Design and Artificial Intelligence
Apart from this example that I just showed, I highlight three other examples that I wanna work with you very quickly. One is on heat exchangers, the other is on lighting fixtures, and the last one is a complex system like on EV Gears. For heat exchangers specifically we’re able to use generative design to come up with heat exchangers that are light weight, that are efficient and beyond what a human can do today. And there are capabilities there, and we’re pushing the limits of these capabilities, and you can see examples of some of the solutions that we’ve been able to develop so far. And what we’ve seen is a fourfold increase in heat rejected per kilogram mass of this product while also reducing the weight of the product by 80%.
Digital Transformation, AI, and the Product Lifecycle
Another example here is our lighting fixtures. First, traditionally, when we design lighting fixtures, we have thermal, electrical, mechanical, optical and manufacturing, critical to quality elements that we need to look at, so it’s a multi-physics problem. So, effectively today it takes us about 16 weeks going through our manual process. The first thing we did is to take this process and digitize it and train an algorithm, it took us 16 weeks, about the same time it takes to manually do this today, to actually train an algorithm that can do this.
Our algorithm and our AI model has up to 90% fidelity. For a new request that came through that we’re looking at, we’re able to design this new request now in two weeks, while effectively cutting down 87% in the design time. This is a plot of where we can look at cost versus temperature, for example, just an example of what our algorithm can do, again, using aPriori, and aPriori supports this in the sense that we can run aPriori programmatically in the back end and create [0:19:50.3] ____ as you see on the chart, where we can select a product out of the many designs that we have that meets our cost as well as our performance requirements.
The last example I’ll give here is autonomous digital design. You may look at some of the components have shown so far as components and just a smaller system, but this is a system of systems, this is a complex system, it’s an EV Gear solution, and effectively what we’ve been able to do so far is cut down the design time by 65%, building… Again, we have high fidelity models that are informing AI algorithms that sits inside an automated workflow and we can cut down design time.
Manufacturing Labor Shortages, Digital Engineering Tools, and Artificial Intelligence
So if you ask me, “Where are we going with all of this?” And I am not going to re-emphasize what Stephanie already presented, but really the Manufacturing Institute and Deloitte, I believe, did a study recently, and they showed that in the US alone there will be over 2 million shortage in manufacturing needs, design manufacturing needs by 2030 with trillion dollar implication. So effectively, if you ask me where we’re heading, where we’re heading is a solution where we can use the resources we need today, and has the outputs that we create using our resources that we have today. Our engineers are not gonna go away, we need them. Right? And we need them to be able to do things faster and create more through-put according to our vision, which is to double the output and the through-put of our new technology introduction, investment.
So if you ask me again, where are we going? I’m showing you here an image of a pitstop for a Formula One car race. For those of you that are fans of this. In 1950 a pitstop took 67 seconds. And this is the interesting part of my job. Where just think about it for a minute, right? 67 seconds for a pitstop, to change tires, to refuel and do some other things. Right? That’s fantastic. And imagine going to the manager of that crew and saying, “Hey, can we cut this down by half?”
The Digital Transformation of Product Development with aPriori
And that’s really my job, my job is going to engineering teams and engineering managers and saying, “How do we cut down your process today by half?” But fast-forward to 1984, the pitstop was 9.3 seconds. That’s more than half reduction. And then you go back to those folks back then in 1984 and say, “Well, can you cut it again by 50%.” But I’m reporting here 2023, and I have a barcode, so if you scan that barcode, I put a video earlier, but I removed it for technical issues, but if you scan that barcode it will give you an example video from, I think, 1950 to 2013, and you will be surprised.
2023, today, a pitstop is 1.87 seconds. Pretty much the time it takes for me to say, with my accent, 1.8 seconds, a pit stop is already done, the tires are changed, it’s back on the race. And if you ask me, that’s where we’re going. And that’s why zero lead time, we continue to cut down, the inefficiencies and continue to accelerate the time to get our products to the market.
So what are some of the things from our organizational standpoint that we’re doing now? We’ve done some POCs, we’ve had success, we have very good excitement across the organization as this is driven top-down as well. Some of the things that are… First, we need the tools, and that’s where tools like aPriori comes in, tools like Ansys simulation come in, tools like ESTECO comes in, a digital thread to support our initiatives. We need knowledge management, we need to capture and manage our knowledge in a format that an algorithm like the one we’re building can use and accelerate the time to deliver. And the last is training and development. So last month, we actually had our first global digital engineering workshop within Eaton with over 450 participants. A lot of presentations across the organization, so pushing and training our workforce so that we can truly accelerate and integrate digital engineering modernization into our DNA as an organization.
So in summary, what I’ve really presented to you here is to state the fact that we’ve been hearing about the term digital engineering, before that it was digitalization. We’ve heard terms like automation in engineering, and the truth is that those technologies are here to stay. I happen to teach in a graduate professional course where I meet students across industries and professionals, and across all organizations, there are elements of automation today, and as we imbibe and bring in these technologies into the way we do engineering, that’s when we start seeing results.
The second is to actually embrace digital technologies, the hype days are over, and today we’re actually looking at how we can integrate them and use them to drive value. We’re not just doing it because it’s fancy or because it’s a buzzword, but because we’re seeing value that comes out of it.
And the last takeaway here, I’m at an aPriori conference, and it’s just the right thing to say here that we partner with organizations like aPriori because they provide us the tools and capabilities, for example, our ability to programmatically run aPriori. Not all tools enable you to programmatically run them in the back-end. Our ability to run simulations in the Cloud and so on. So these are capabilities that aPriori provides us that allows us to truly partner with them at Eaton. Thank you so much.